What covariariance and correlation are. Falls in any particular range or discrete set of values specified for that variable is defined as the joint probability distribution for a b.

Joint Probability Distributions And Random Samples Ppt Video Online Download
Random variables characterized by a joint probability distribution function jpdf defined in a bayesian framework are generally sampled with markov chain monte carlo mcmc.
Random sample joint probability distribution. Suppose one has a box of ten balls four are white three are red and three are black. 62 joint probability mass function. To begin the discussion of two random variables we start with a familiar example.
Given random variables that are defined on a probability space the joint probability distribution for is a probability distribution that gives the probability that each of falls in any particular range or discrete set of values specified for that variable. Shao and anis younes. Sampling from a box.
Random variables characterized by a joint probability distribution function jpdf defined in a bayesian framework are generally sampled with markov chain monte carlo mcmc. The probability distribution that gives the probability that each of a b. First note that by the assumption beginequation nonumber fyxyx left beginarrayl l frac12x quad x leq y leq x quad.
Visualizing multiple variablesjoint probability distributions. 1 joint probability distributions recall that a basic probability distribution is dened over a random variable and a random variable maps from the sample space to the real numbers r. The latter can be computationally demanding when the number of variables is high.
Random sampling from joint probability distributions defined in a bayesian framework. Support of x is equal to x1 x2 and so on xm. Now let us introduce the definition of joint probability distribution.
We will write it in the following way. Let a b be the random variables which are defined on a probability space. In the case of only two random variables this is called a bivariate distribution but the concept generalizes to any.
The latter can be. Then we say xy are jointly continuous with joint probability density function f. A bit more about variance.
Building a joint distribution. To capture this distinction we have to introduce the notion of joint probability. Suppose x is the true approval rating this is a model of course and y is the number of voters out of a simple random sample of size 150 who approve.
This example is one. Let us consider two random variables x and y let us assume that x takes value x1 and so on xn.
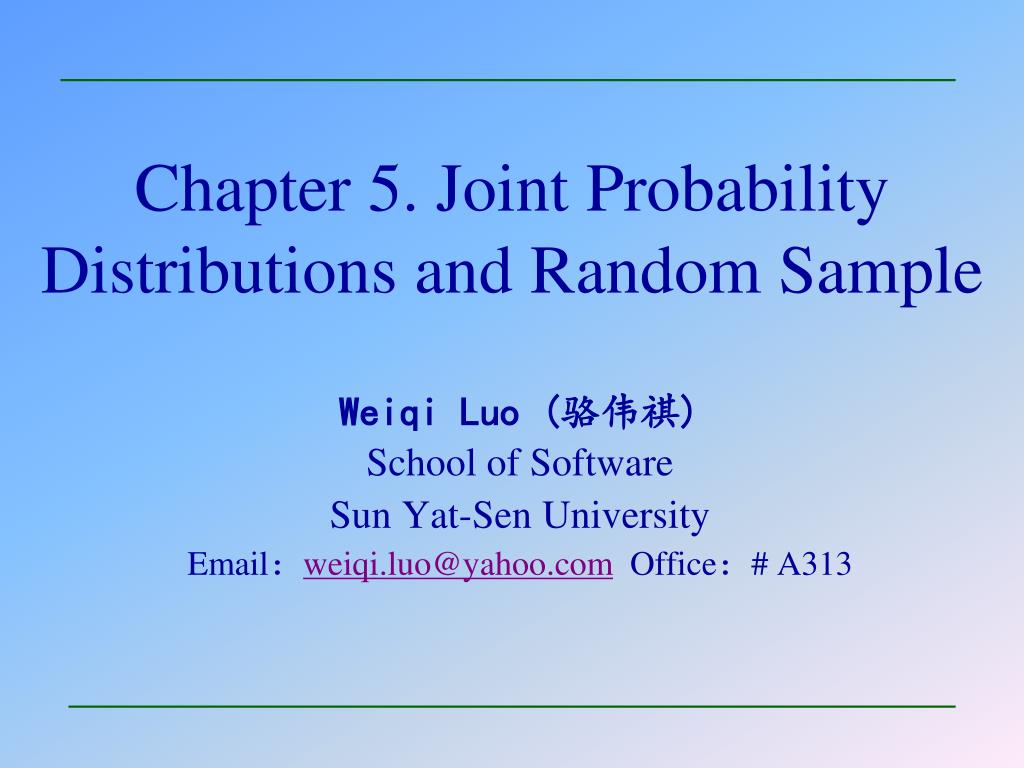
Ppt Chapter 5 Joint Probability Distributions And Random Sample Powerpoint Presentation Id 5443643

Joint Probability Distributions And Random Samples Ppt Video Online Download
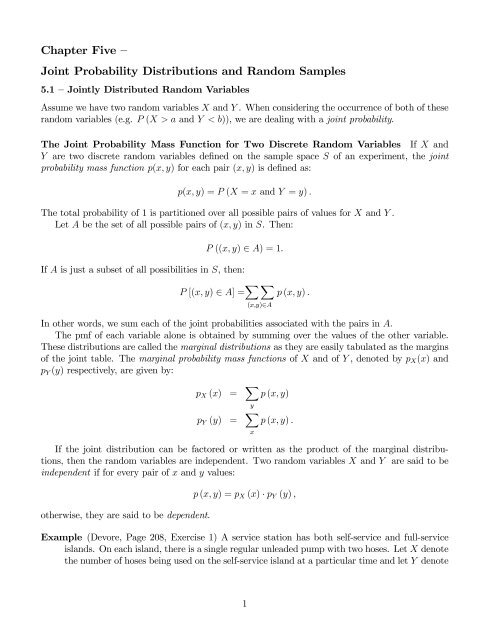
Chapter Five A Joint Probability Distributions And Random Samples

Joint Probability Distribution Wikipedia
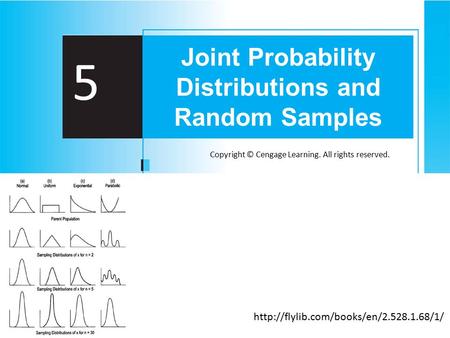
Joint Probability Distributions And Random Samples Ppt Download

Joint Probability Distribution Wikipedia
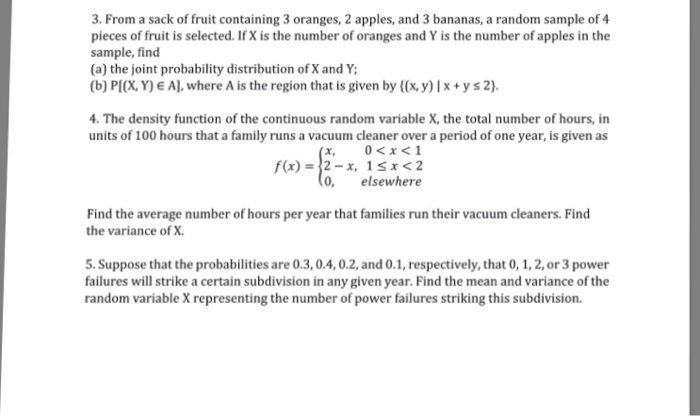
Solved From A Sack Of Fruit Containing 3 Oranges 2 Apple Chegg Com

2
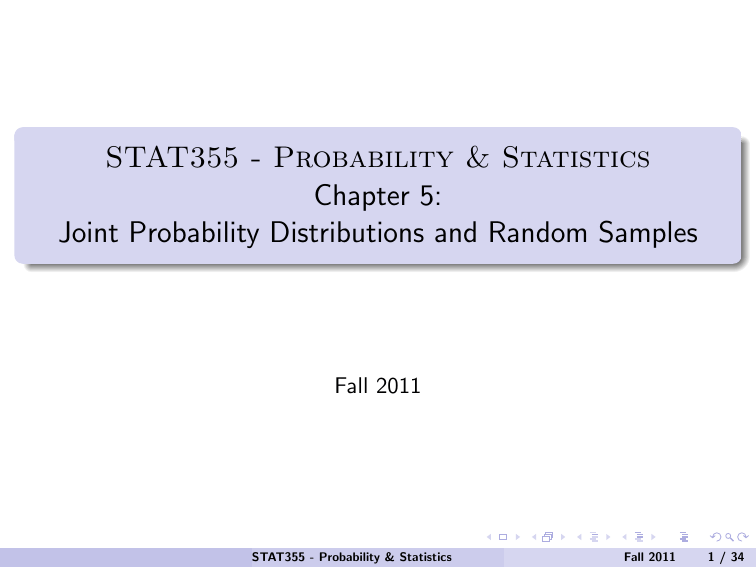
Stat355 Probability Statistics Chapter 5 Joint Probability
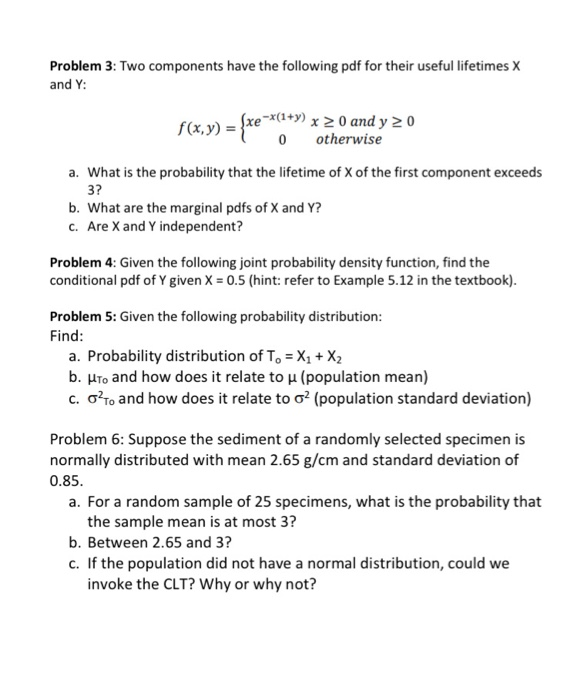
Solved Problem 3 Two Components Have The Following Pdf F Chegg Com
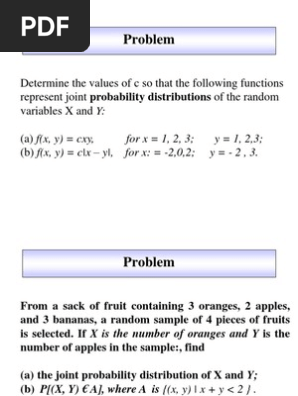
Joint Distributions Random Variable Variance

Http Ccrgpages Rit Edu Whelan Courses 2010 4wi 1016 345 Notes05 Pdf

Joint Probability Distributions And Random Samples Ppt Download
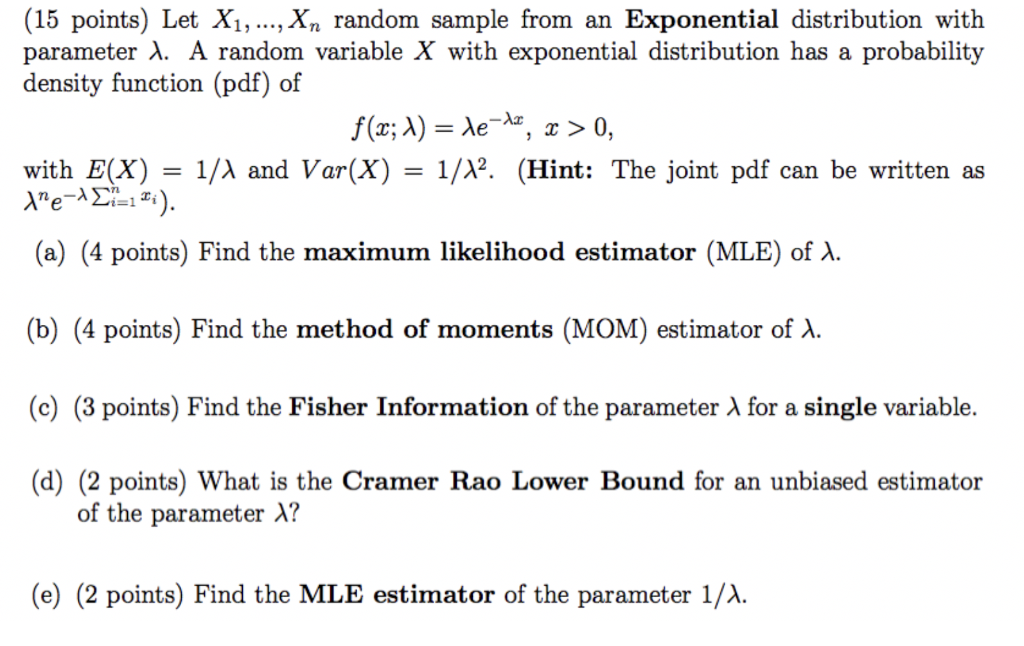
Solved 15 Points Let X1 Xn Random Sample From An Chegg Com

5 1 2 Pptx Chapter 5 Joint Probability Distributions And Random Samples 5 1 Jointly Distributed Random Variables 5 2 Expected Values Covariance And Course Hero
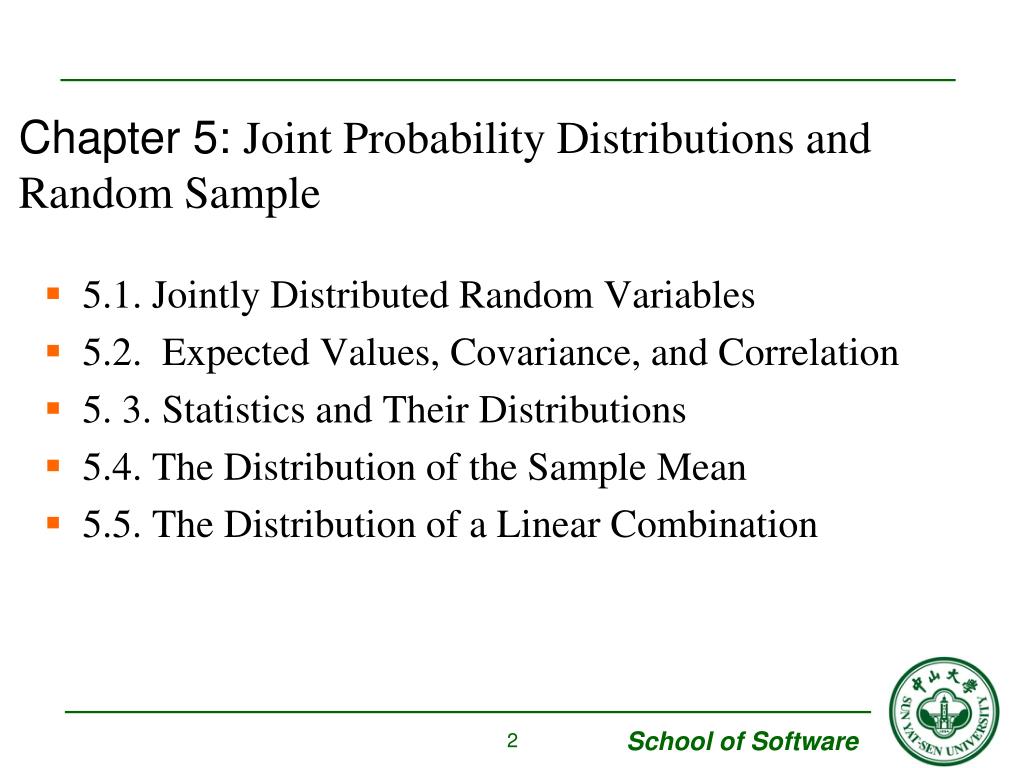
Ppt Chapter 5 Joint Probability Distributions And Random Sample Powerpoint Presentation Id 5443643

St 371 Viii Theory Of Joint Distributions Pdf Free Download
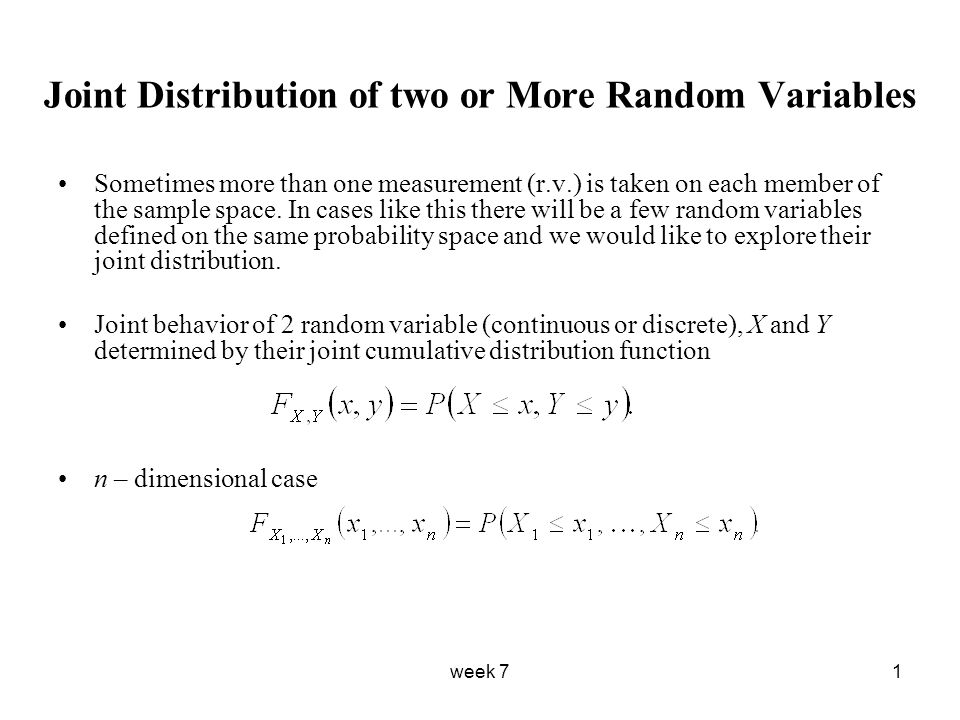
Joint Distribution Of Two Or More Random Variables Ppt Video Online Download

Chapter 5 Joint Probability Distributions And Random Samples 5 Joint Probability Distributions And Random Samples 5 1 Jointly Distributed Random Course Hero
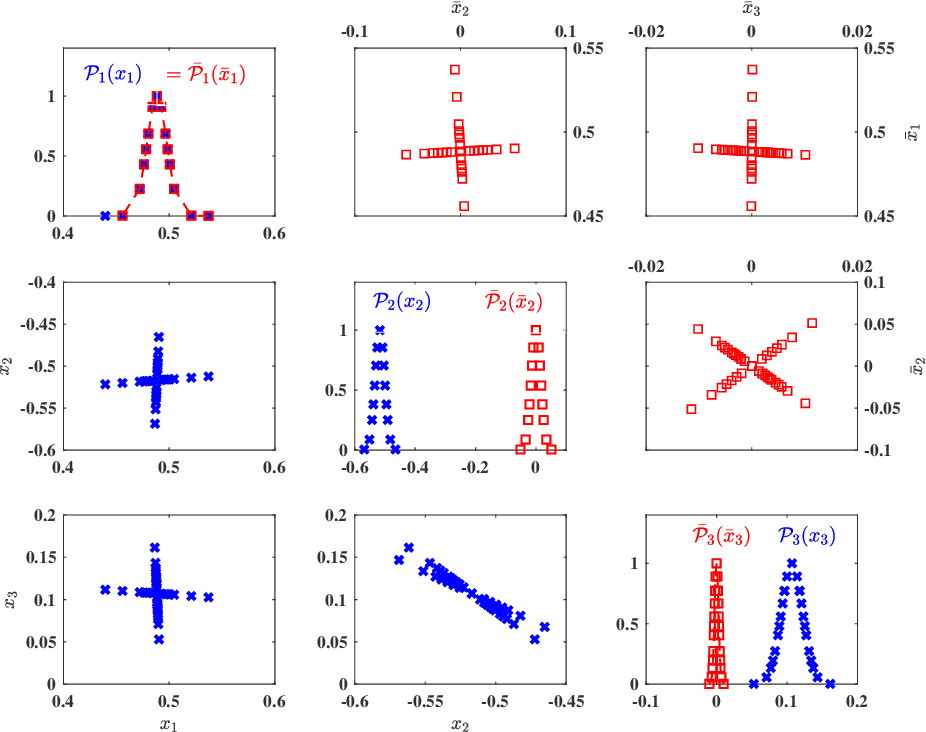
Pdf Random Sampling From Joint Probability Distributions Defined In A Bayesian Framework Semantic Scholar

Lesson 17 Distributions Of Two Discrete Random Variables

A Gentle Introduction To Probability Density Estimation

Https Encrypted Tbn0 Gstatic Com Images Q Tbn And9gcsnmneldet5s Yx Veyk2oamjfxrxbgyb11wnpppmpxnmlyoss2 Usqp Cau
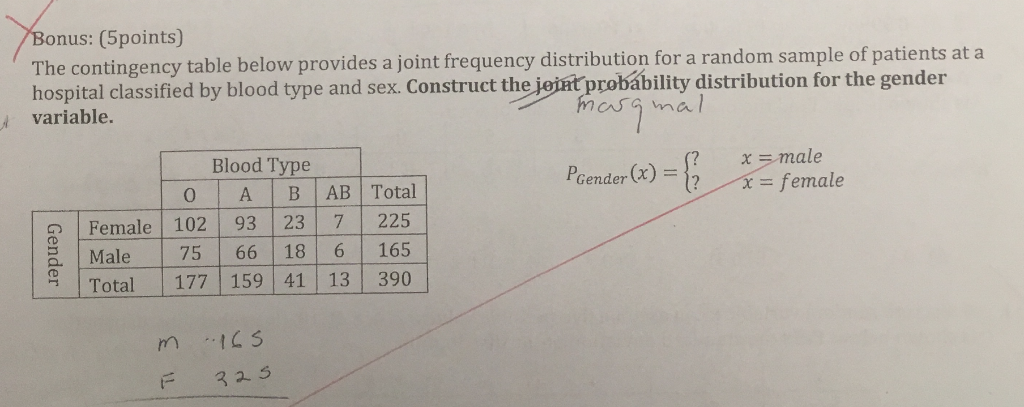
Solved Onus 5points The Contingency Table Below Provid Chegg Com

Ppt 5 1 Jointly Distributed Random Variables Powerpoint Presentation Id 3141355
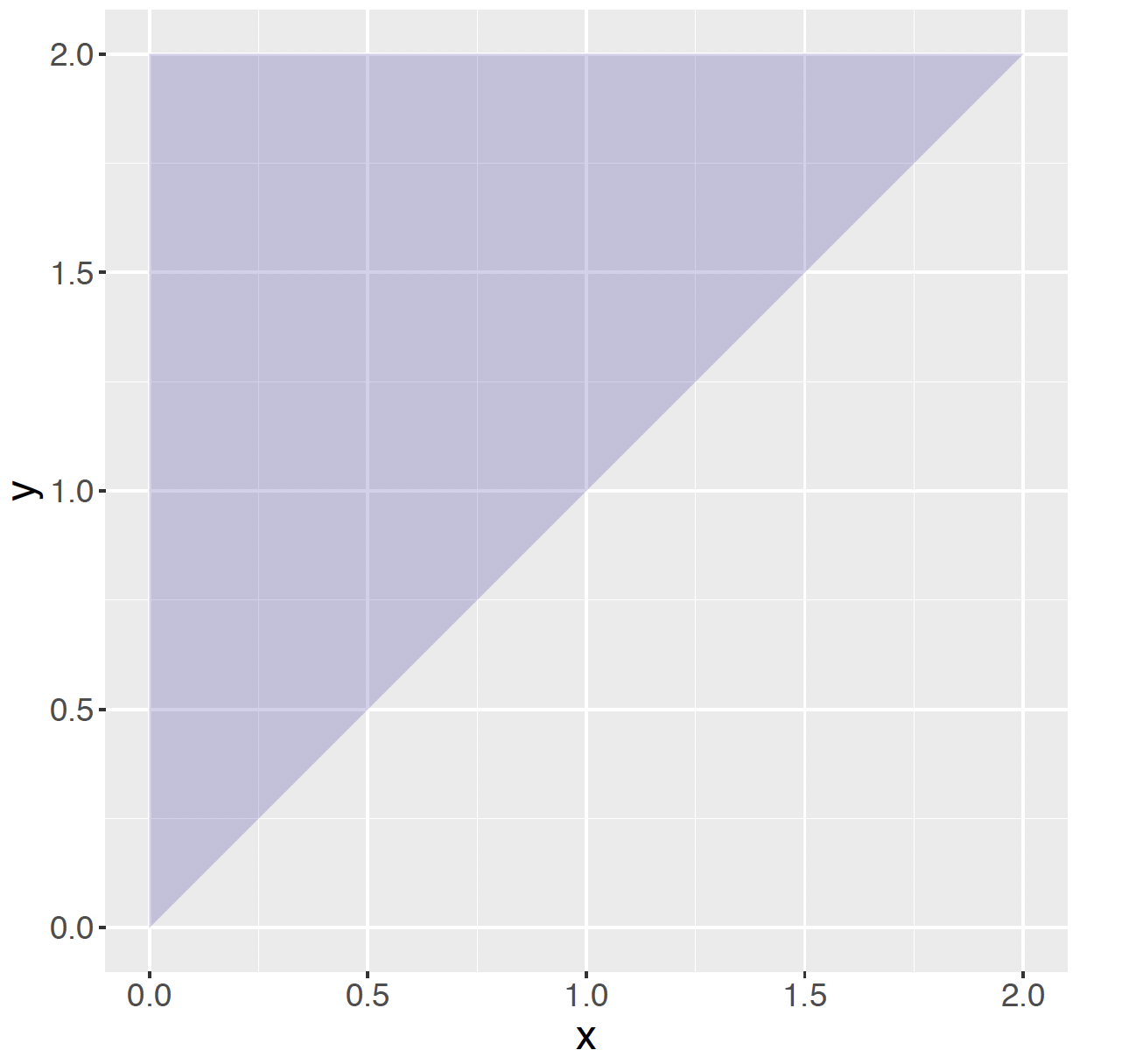
Chapter 6 Joint Probability Distributions Probability And Bayesian Modeling

Deep Learning Book Series 3 4 And 3 5 Marginal And Conditional Probability By Hadrien Jean Towards Data Science
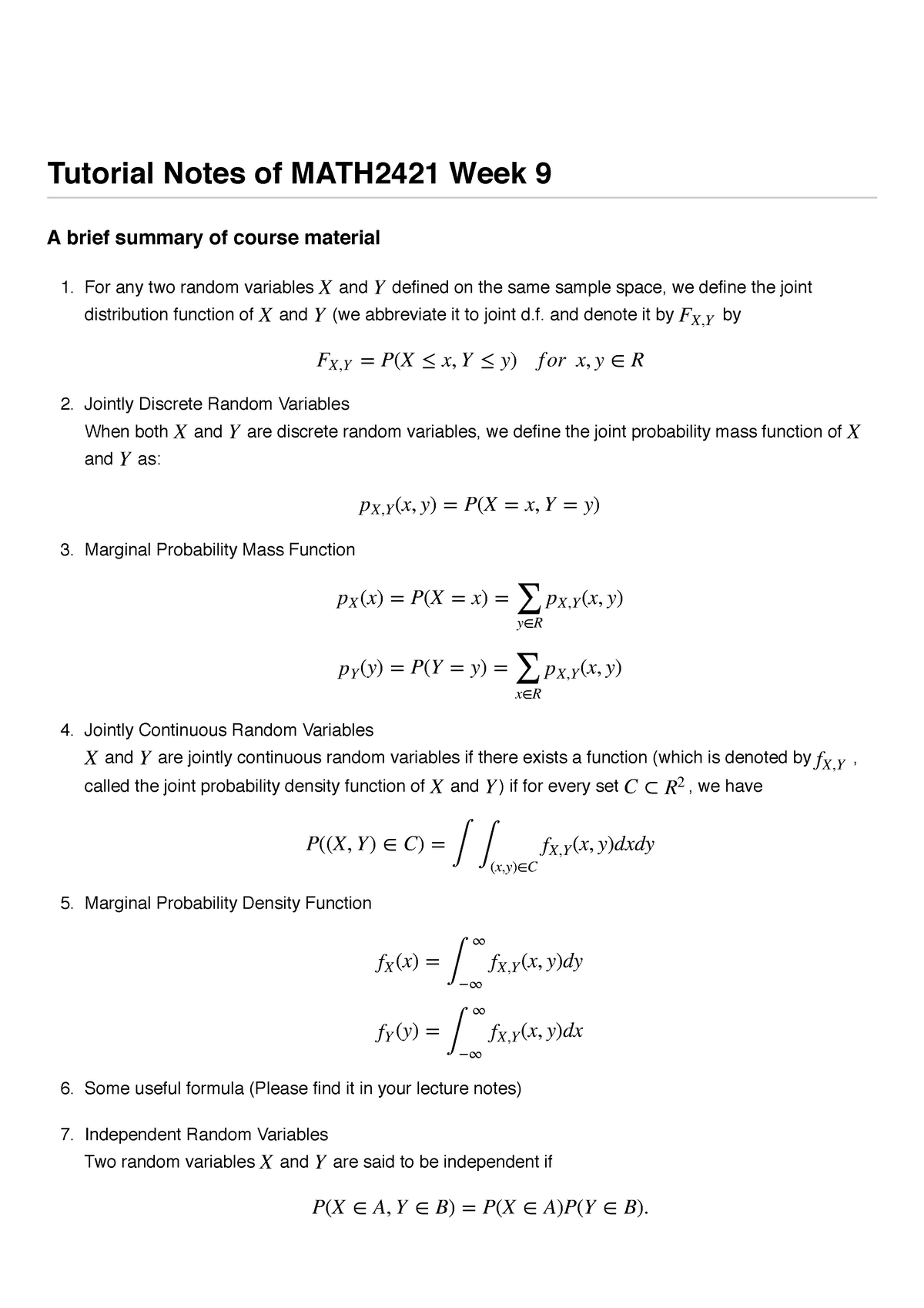
Ta Notes Week9 Bao Zhigang Tutorial Notes Of Math2421 Week Brief Summary Of Studocu
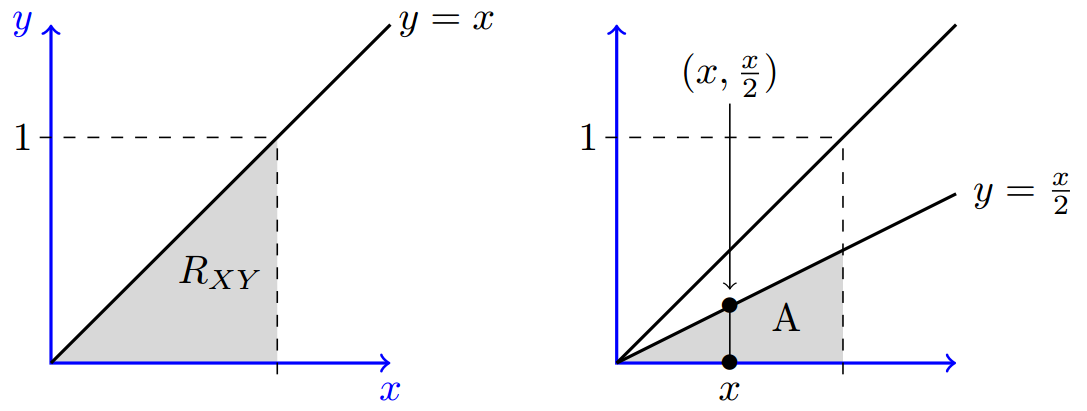
Joint Probability Density Function Joint Continuity Pdf
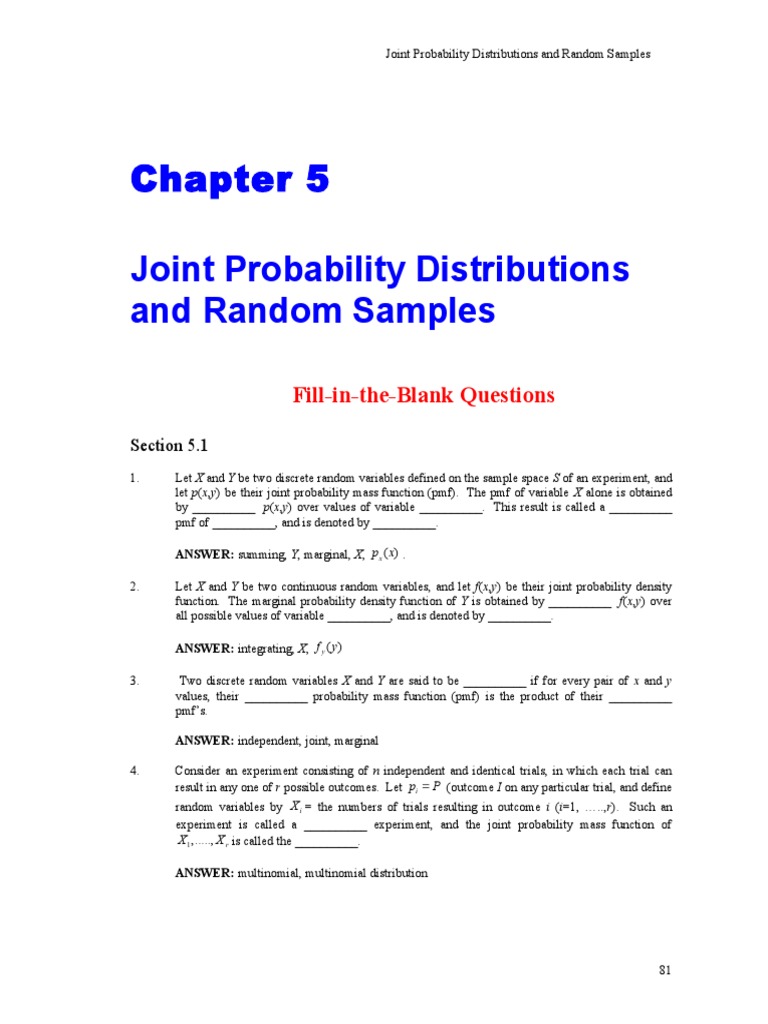
Chapter 5 Correlation And Dependence Mean
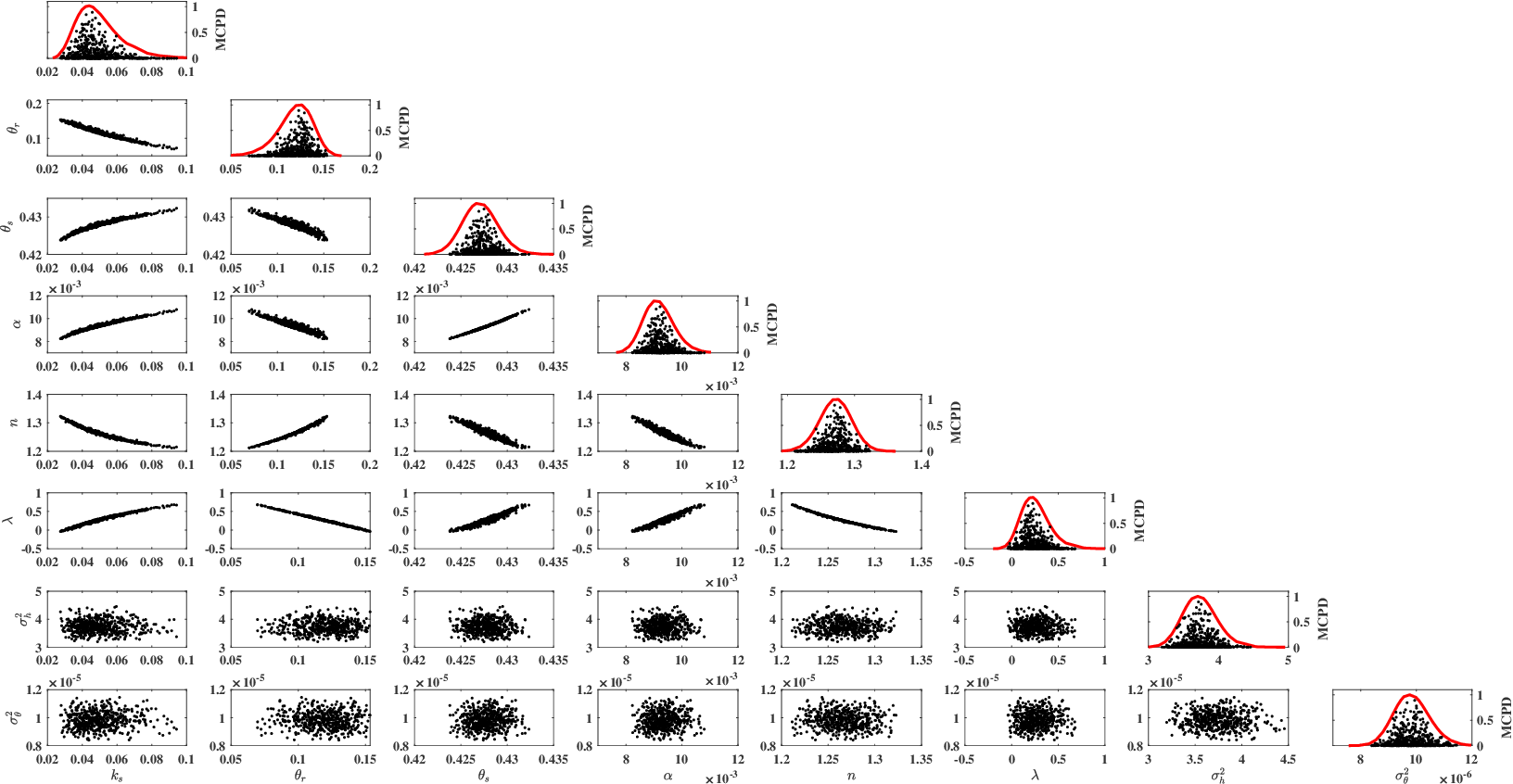
Pdf Random Sampling From Joint Probability Distributions Defined In A Bayesian Framework Semantic Scholar

Y 1 4 Z 2 Section 2 20 Points Each 1 From A Sack Of Fruit Containing 3 Oranges Course Hero
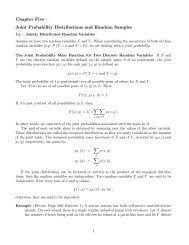
Bivariate Or Joint Probability Distributions
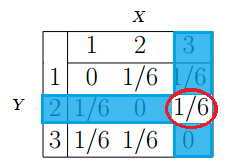
Joint Probability And Joint Distributions Definition Examples Statistics How To
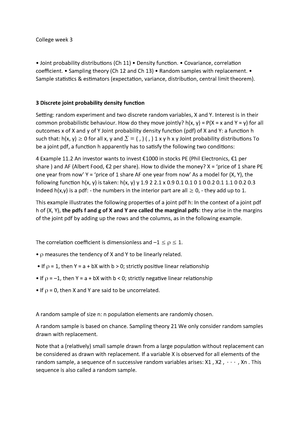
Aantekeningen College 3 Stat Studeersnel
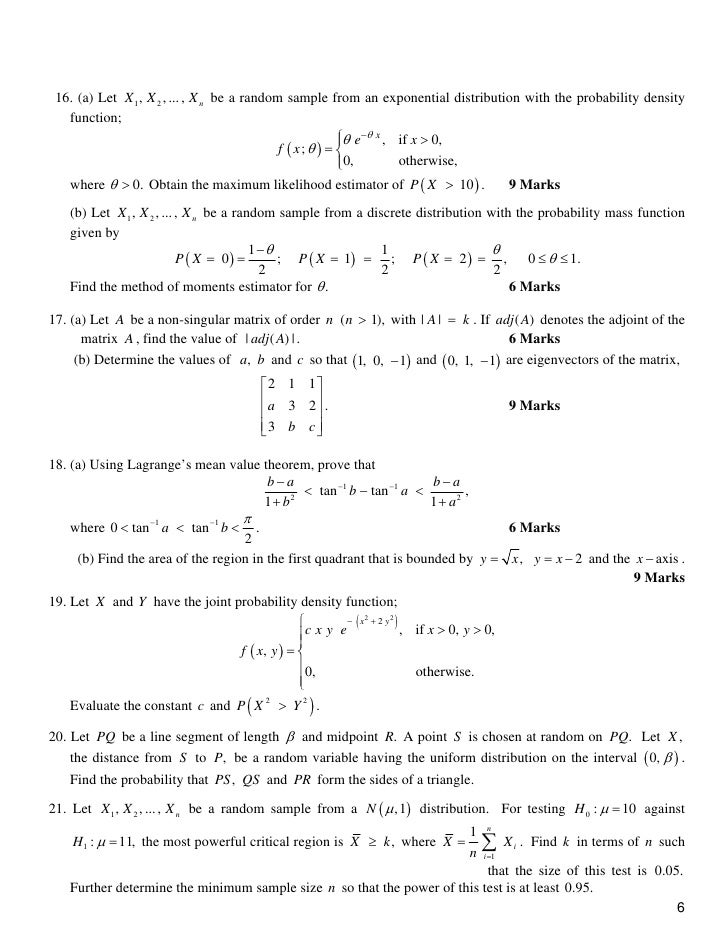
Jam 2006 Test Papers Mathematical Statistics

Http Erho Weebly Com Uploads 2 7 8 4 27841631 Le 1 Review Exercises 2 Pdf
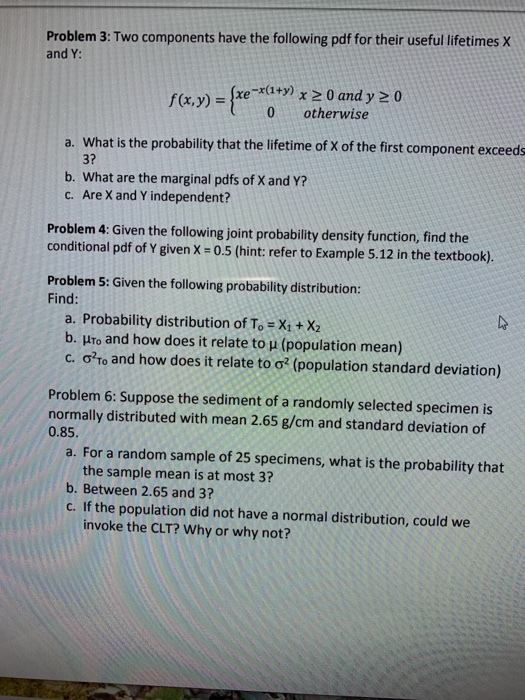
Solved Problem 3 Two Components Have The Following Pdf F Chegg Com

Fegyverneki Sandor Probability Theory And Mathematical Pdf Free Download

Order Statistic Wikipedia

Http Www Domas Store Download Php File Final Sp14 Pdf

Http Webstor Srmist Edu In Web Assets Srm Mainsite Files 2018 Twodimensionalrandomvariable Unit 2 Pdf
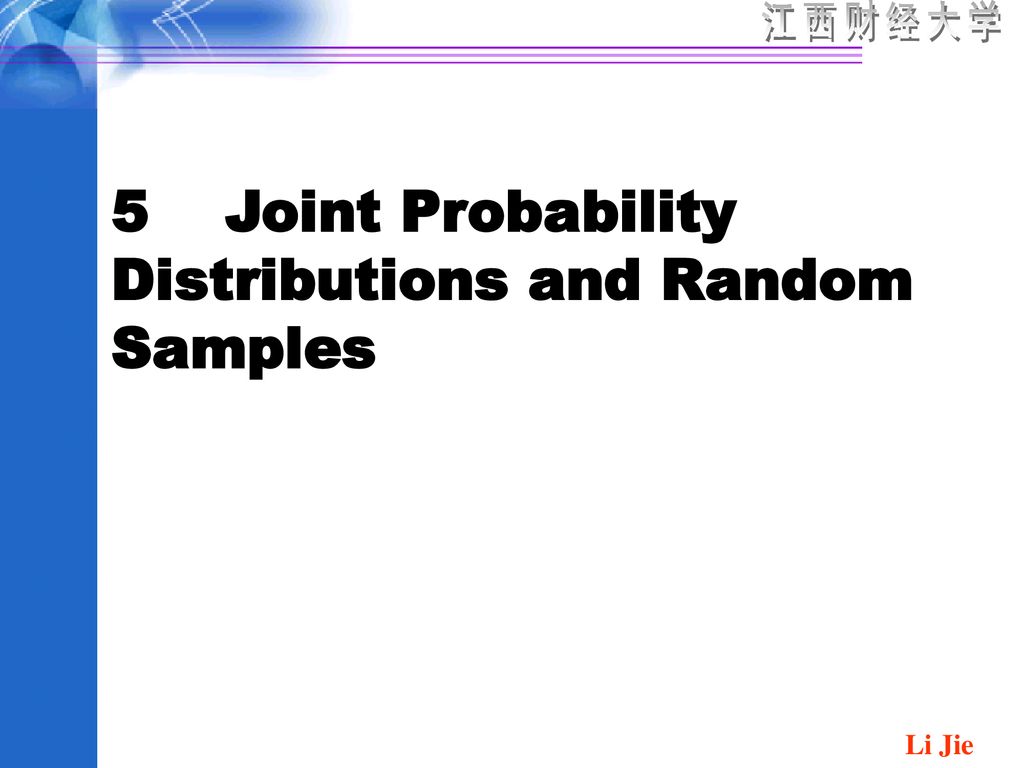
5 Joint Probability Distributions And Random Samples Ppt Download

Https Files Oakland Edu Users Qu Web Sta226winter2010 Ch05slide Pdf
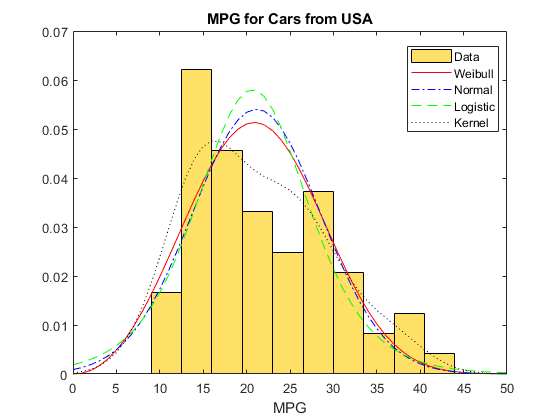
Probability Distributions Matlab Simulink

A Gentle Introduction To Probability Density Estimation

Joint Probability Distribution In Quantitative Techniques For Management Tutorial 12 January 2021 Learn Joint Probability Distribution In Quantitative Techniques For Management Tutorial 10064 Wisdom Jobs India

Https Encrypted Tbn0 Gstatic Com Images Q Tbn And9gcq 1h Bxbkuip1ahc Llt1ek3cc7khyvdswxfw0jvjwgyaaxjit Usqp Cau
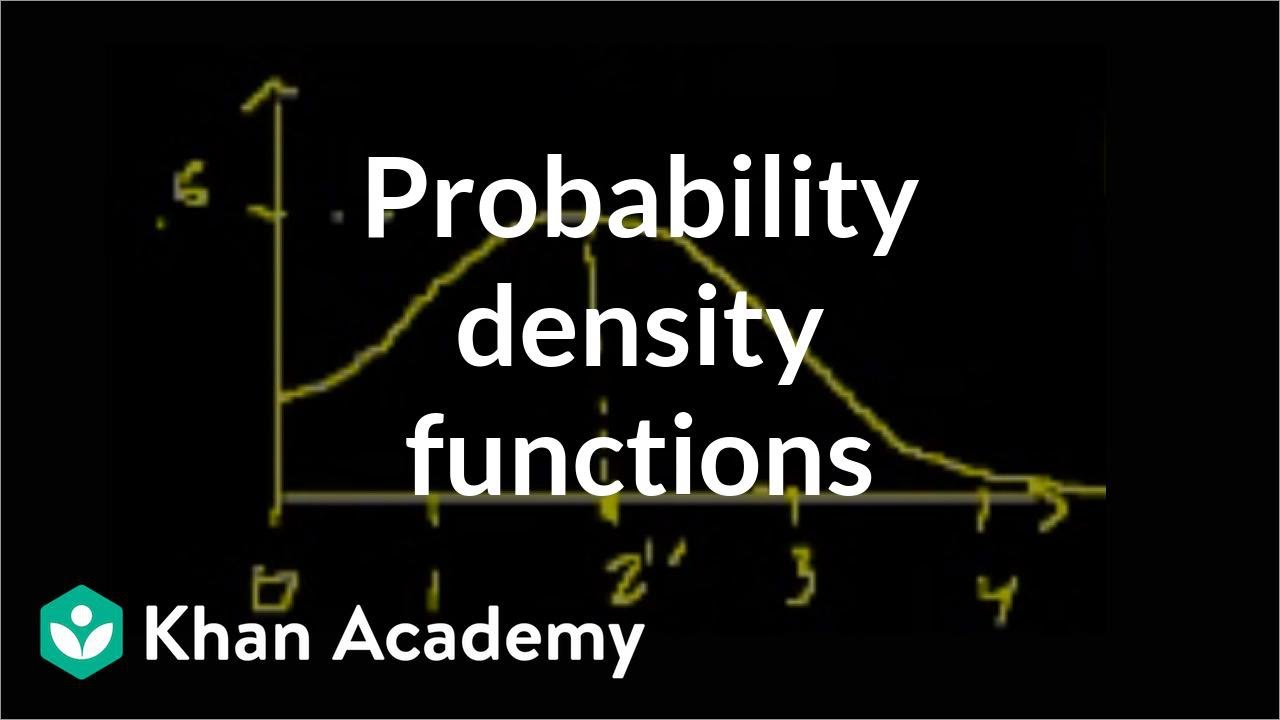
Probability Density Functions Video Khan Academy
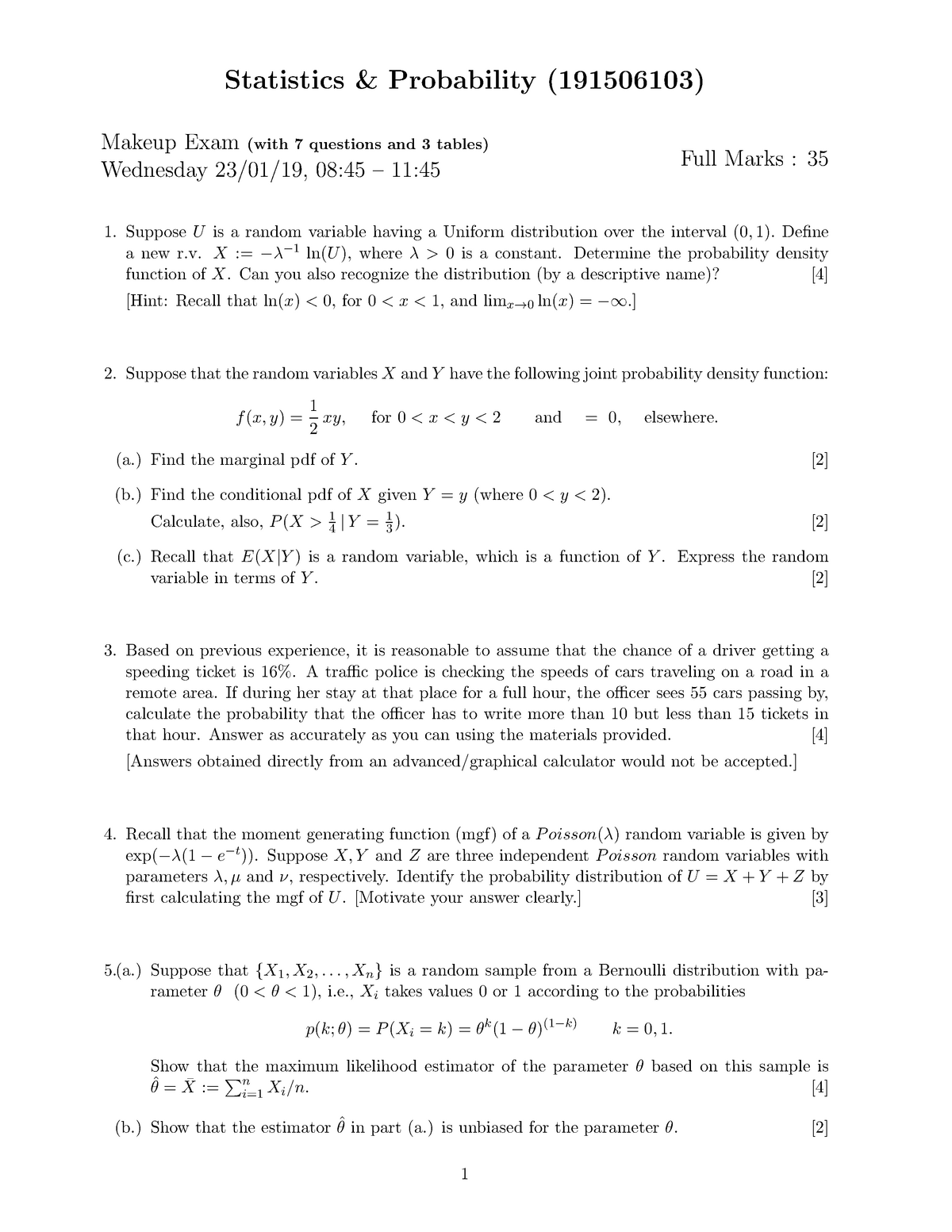
Tentamen 6 Februari 2019 Vragen 191506103 Studocu

Https Csula Development Preview Com Sites Default Files Groups Department 20of 20mathematics Probabilitycomps Probabilitys17 Pdf

Probability And Random Variable Powerpoint Slides

Joint Probability Density Function Of A Sample Of A Normal Distribution Mathematics Stack Exchange
/JointProbabilityDefinition2-fb8b207be3164845b0d8706fe9c73b01.png)
Joint Probability Definition
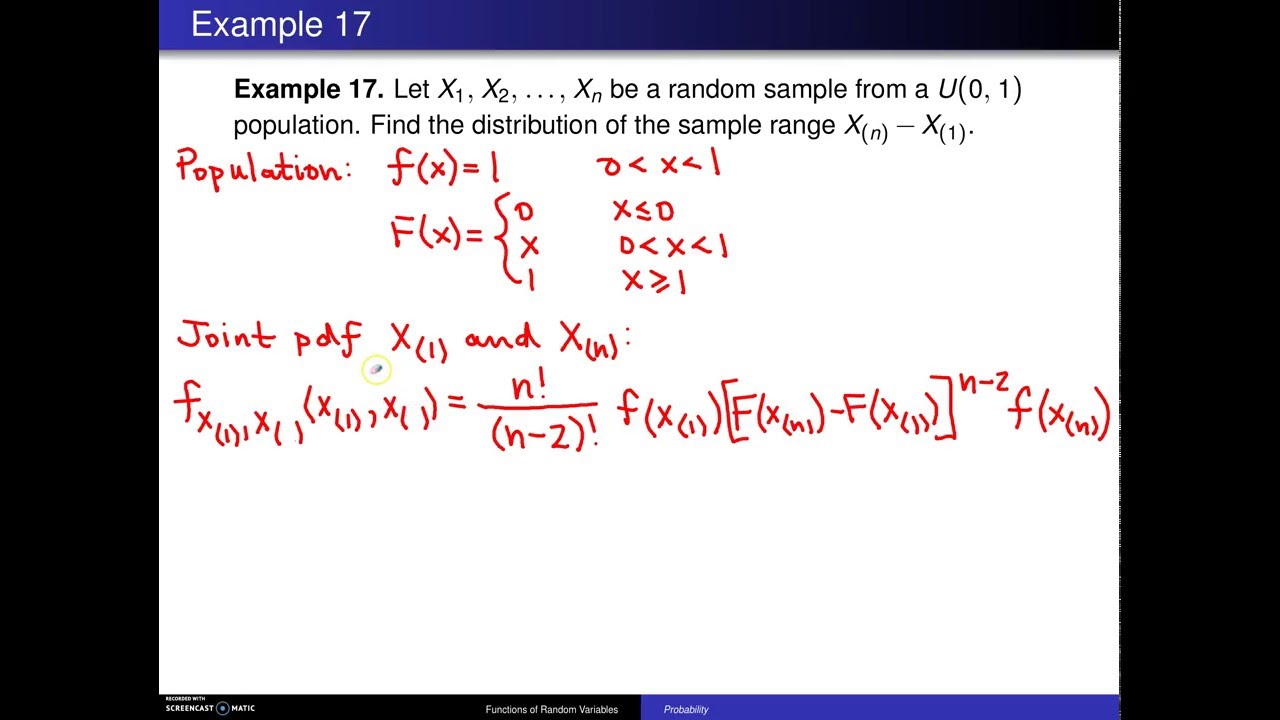
Order Statistics Example 7 Youtube
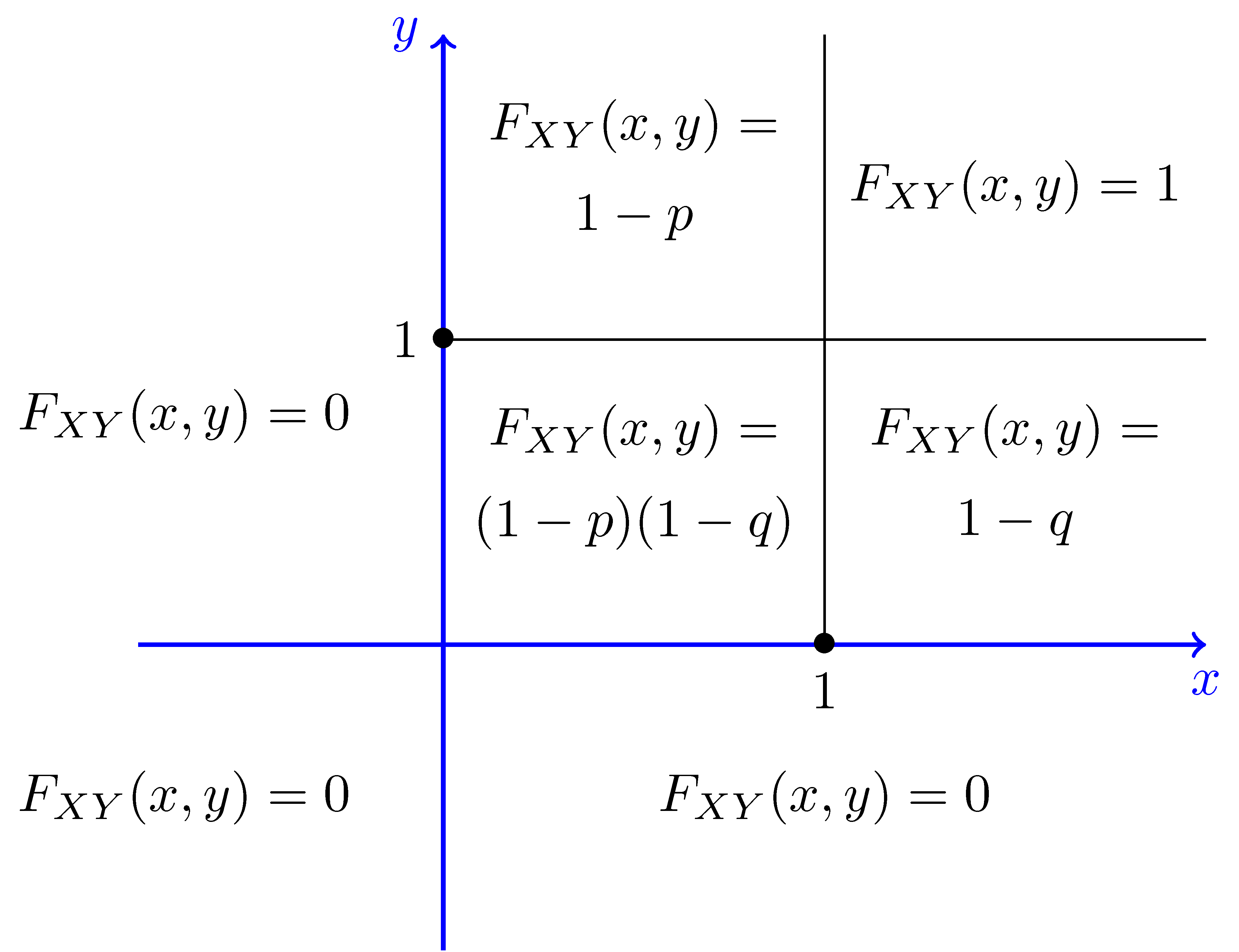
Joint Cumulative Distributive Function Marginal Pmf Cdf

20 Questions With Answers In Joint Probability Distribution Science Topic
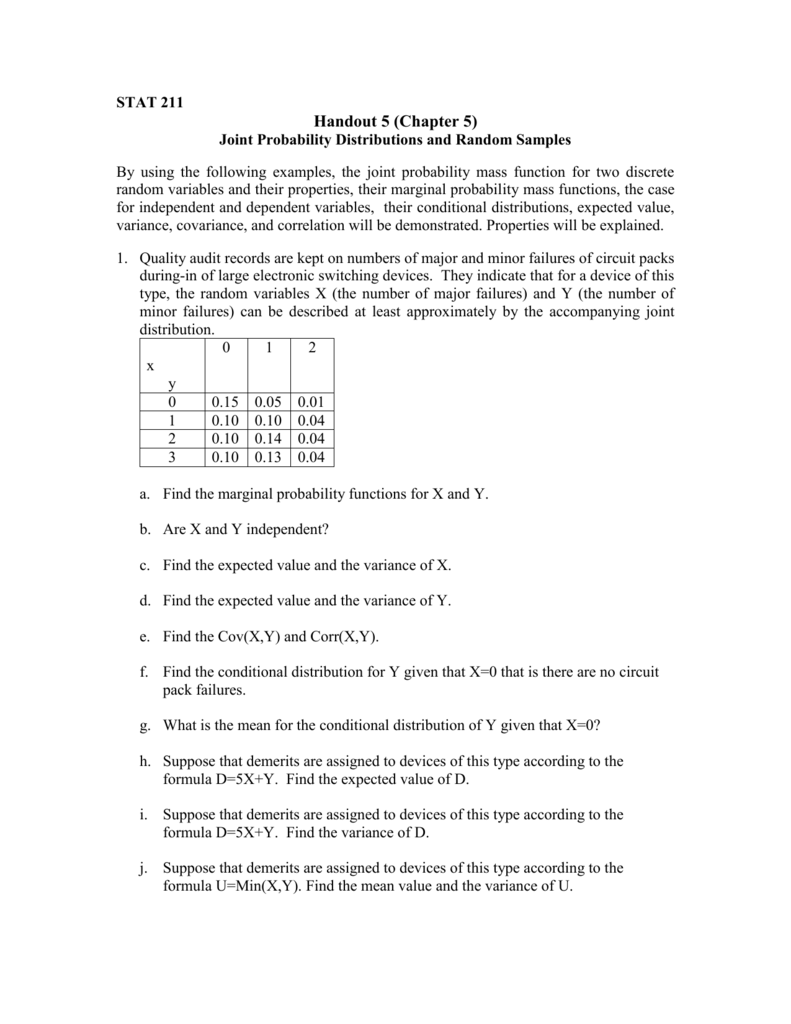
Suppose That A Pair Of Random Variables Have The Same Joint

2

2

Https Www Colorado Edu Amath Sites Default Files Attached Files Hw9 5 Pdf

Ppt Chapter 5 Joint Probability Distributions And Random Sample Powerpoint Presentation Free To View Id 455523 Odg3z

Joint Probability An Overview Sciencedirect Topics

Tb Chapter5 Joint Probability Distributions And Random Samples Chapter 5 Joint Probability Distributions And Random Samples Fill In The Blank Course Hero

Http Oldwww Unibas It Utenti Dinardo Ascsq Pdf

Joint Probability Distributions
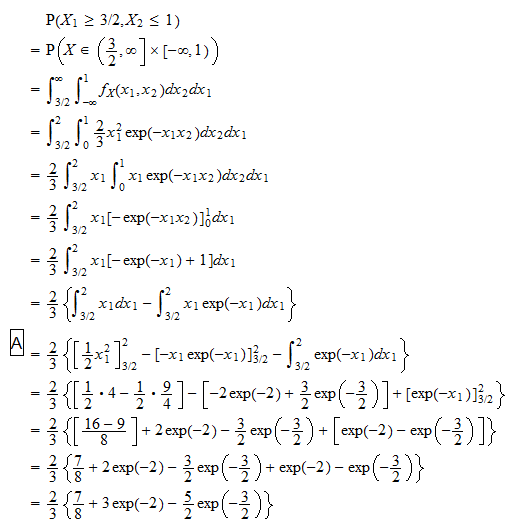
Joint Probability Density Function
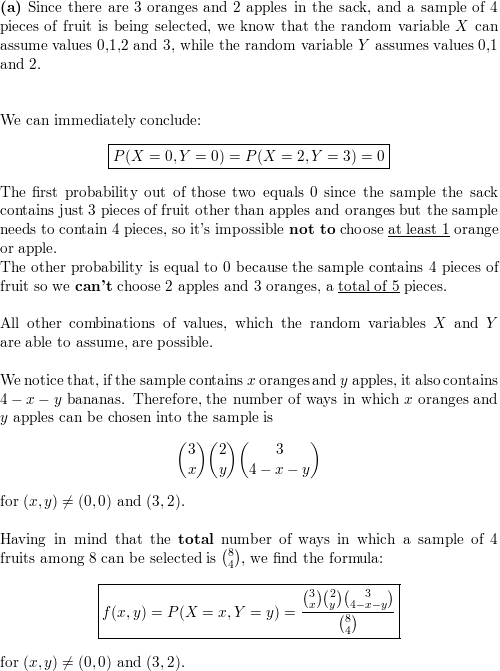
From A Sack Of Fruit Containing 3 Oranges 2 Apples And 3 Bananas A Random Sample Of 4 Pieces Of Fruit Is Selected If X Is The Number Of Oranges And Y
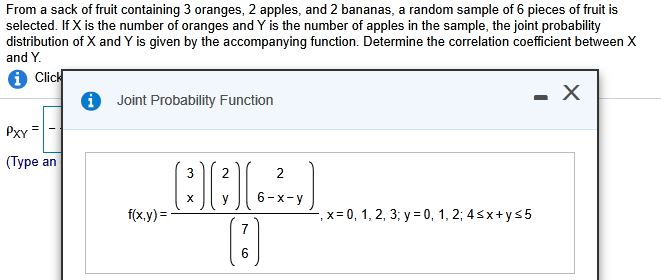
Solved From A Sack Of Fruit Containing 3 Oranges 2 Apple Chegg Com
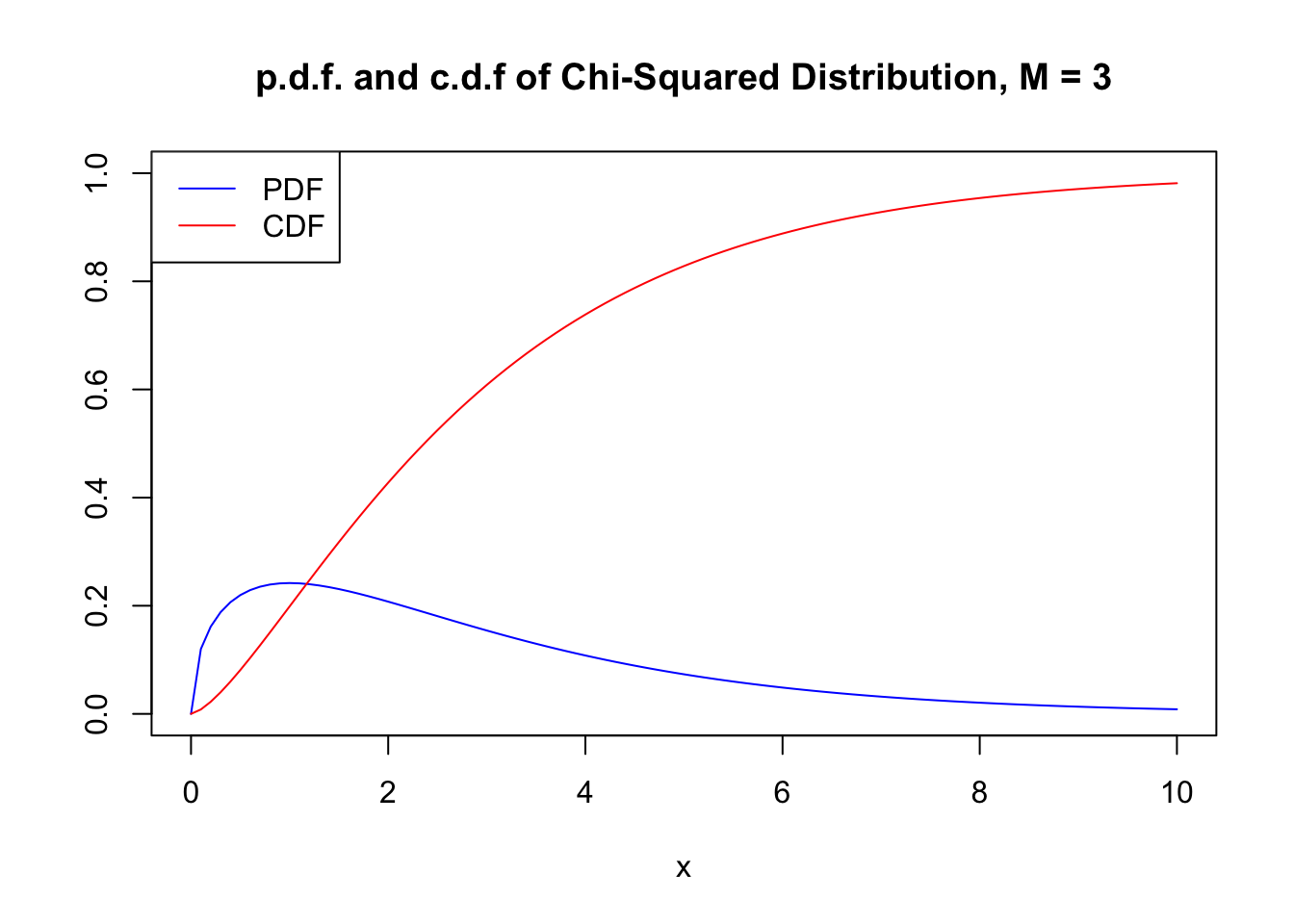
2 1 Random Variables And Probability Distributions Introduction To Econometrics With R

Http Www Nuigalway Ie Maths Jh Ma237 Handout10 Pdf

Two Dimensional Random Variables

Https Encrypted Tbn0 Gstatic Com Images Q Tbn And9gcsnmneldet5s Yx Veyk2oamjfxrxbgyb11wnpppmpxnmlyoss2 Usqp Cau
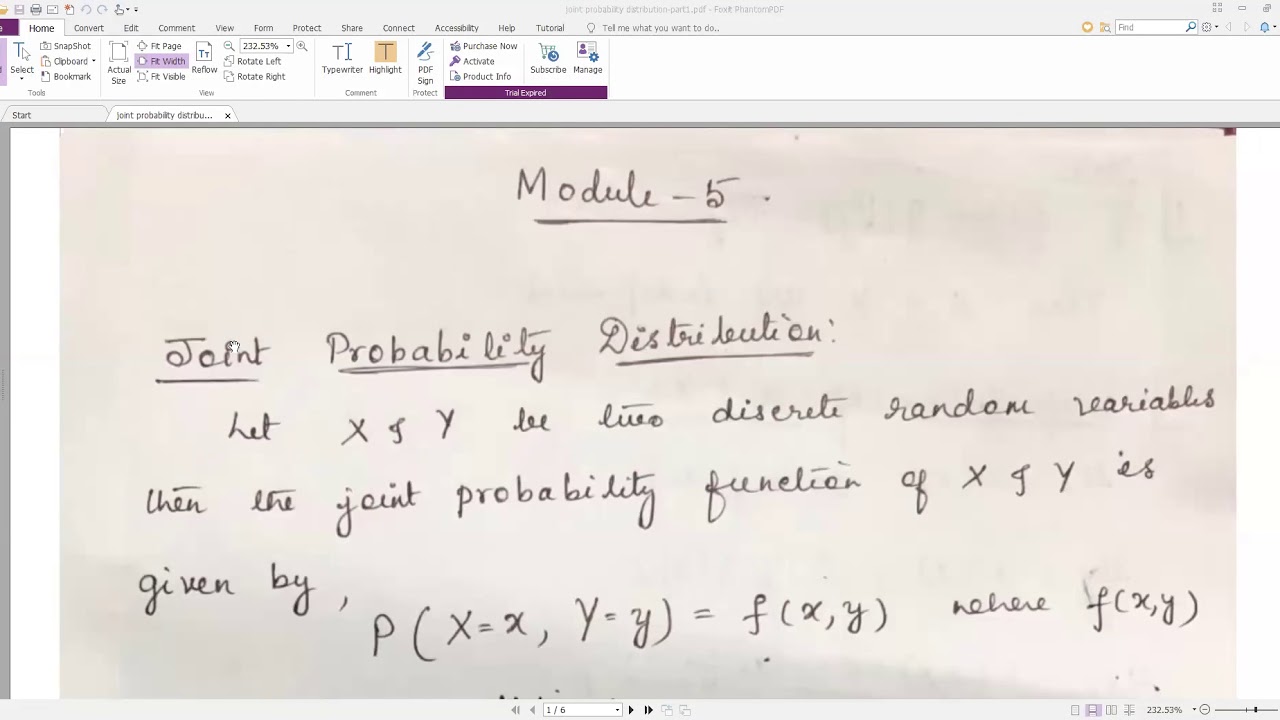
Module5 Joint Probability Distribution And Sampling Theory Iv Sem Youtube

Https Www Jstor Org Stable 2334509

Probability And Random Variable Powerpoint Slides
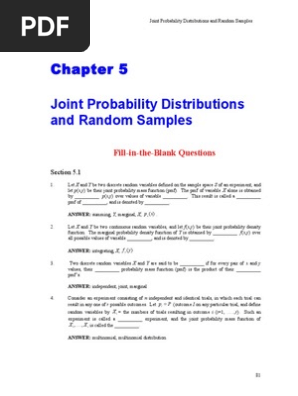
Chapter 5 Correlation And Dependence Mean

Marginal Density Functions Of Gaussian Distributed Random Variables X Download Scientific Diagram

Fegyverneki Sandor Probability Theory And Mathematical Pdf Free Download

Uor 7 1 3

Https Www Stt Msu Edu Users Makagon 351 5 1 Pdf
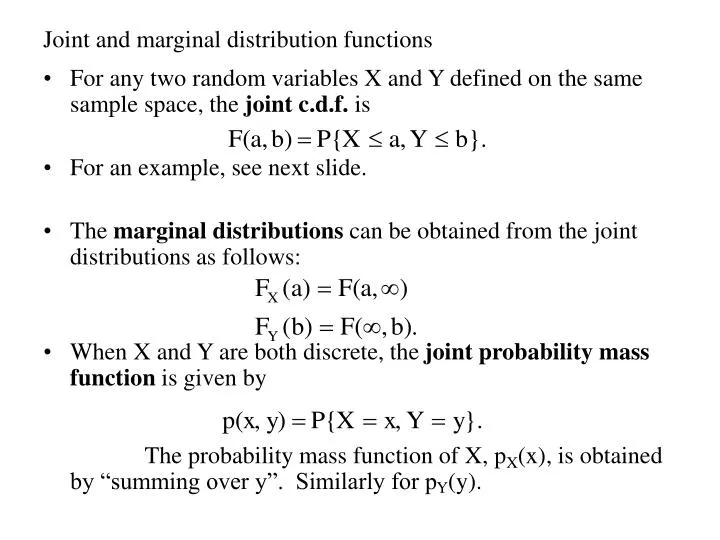
Ppt Joint And Marginal Distribution Functions Powerpoint Presentation Id 175578

Normal Distribution And Joint Probability Distribution

2

Http Math Arizona Edu Jwatkins Joint Pdf
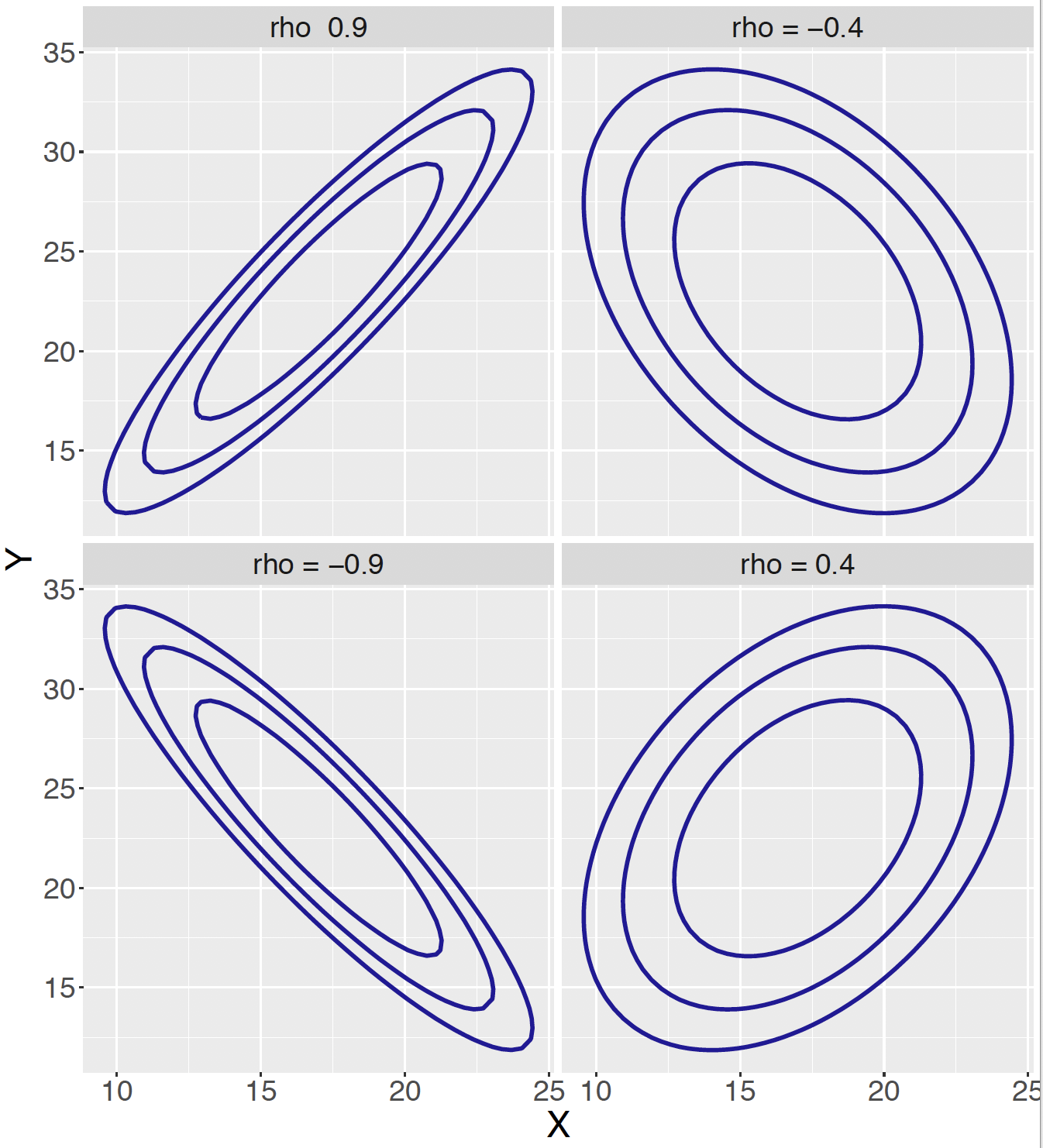
Chapter 6 Joint Probability Distributions Probability And Bayesian Modeling
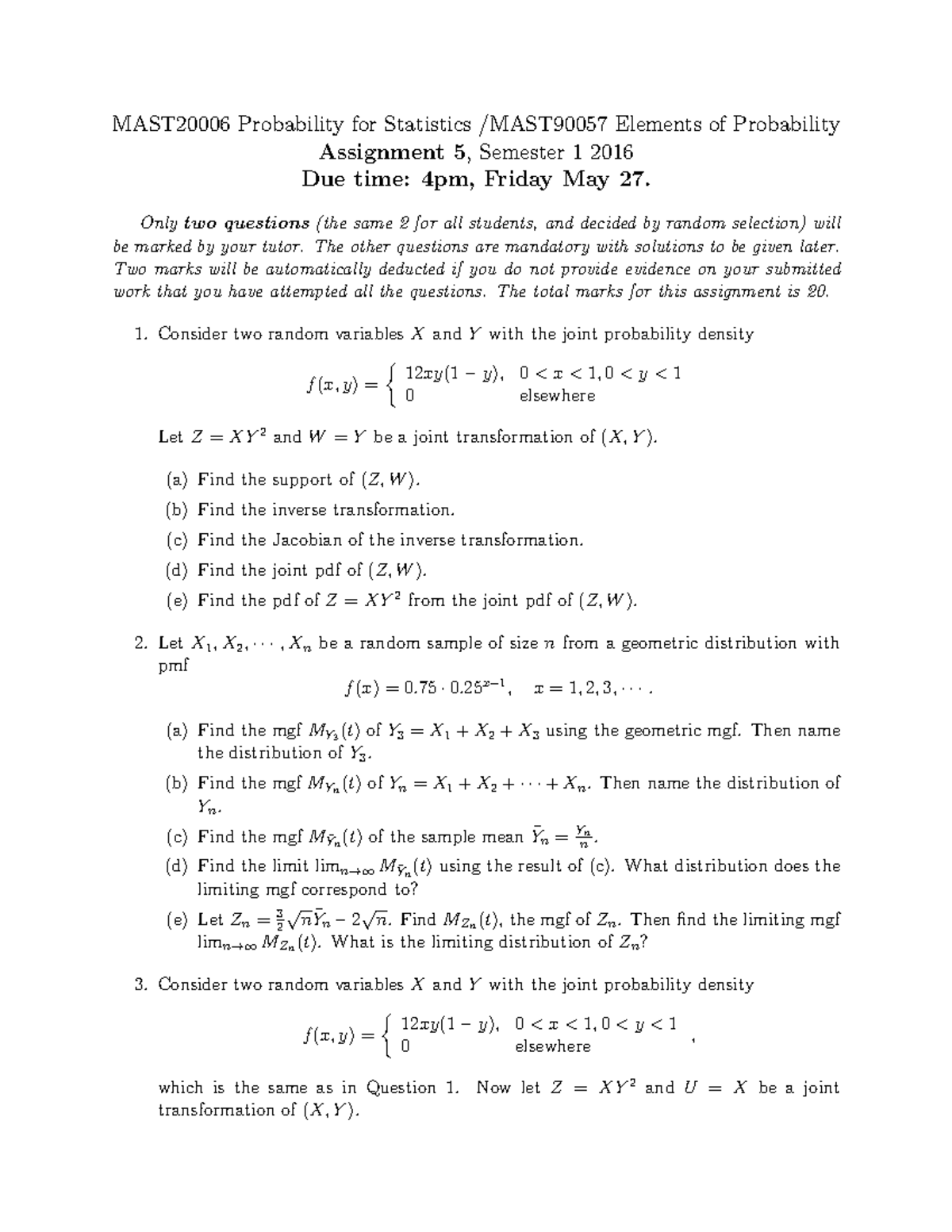
Seminar Assignments Mast20006 Studocu

Lesson 17 Distributions Of Two Discrete Random Variables

Two Dimensional Random Variables
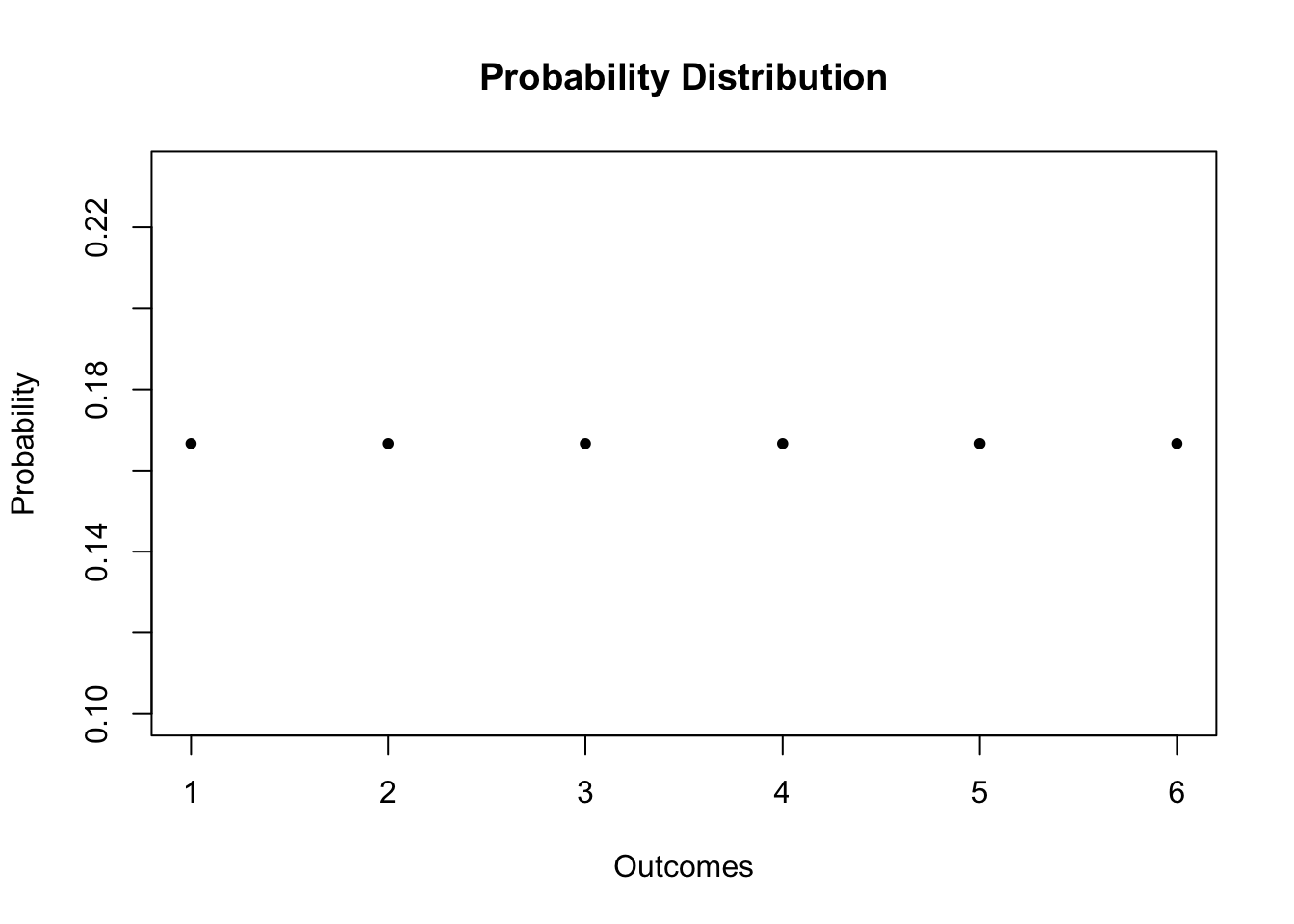
2 1 Random Variables And Probability Distributions Introduction To Econometrics With R

Http Faculty Atu Edu Mfinan 3153 Section26 Pdf

Stat355 Probability Statistics Chapter 5 Joint Probability
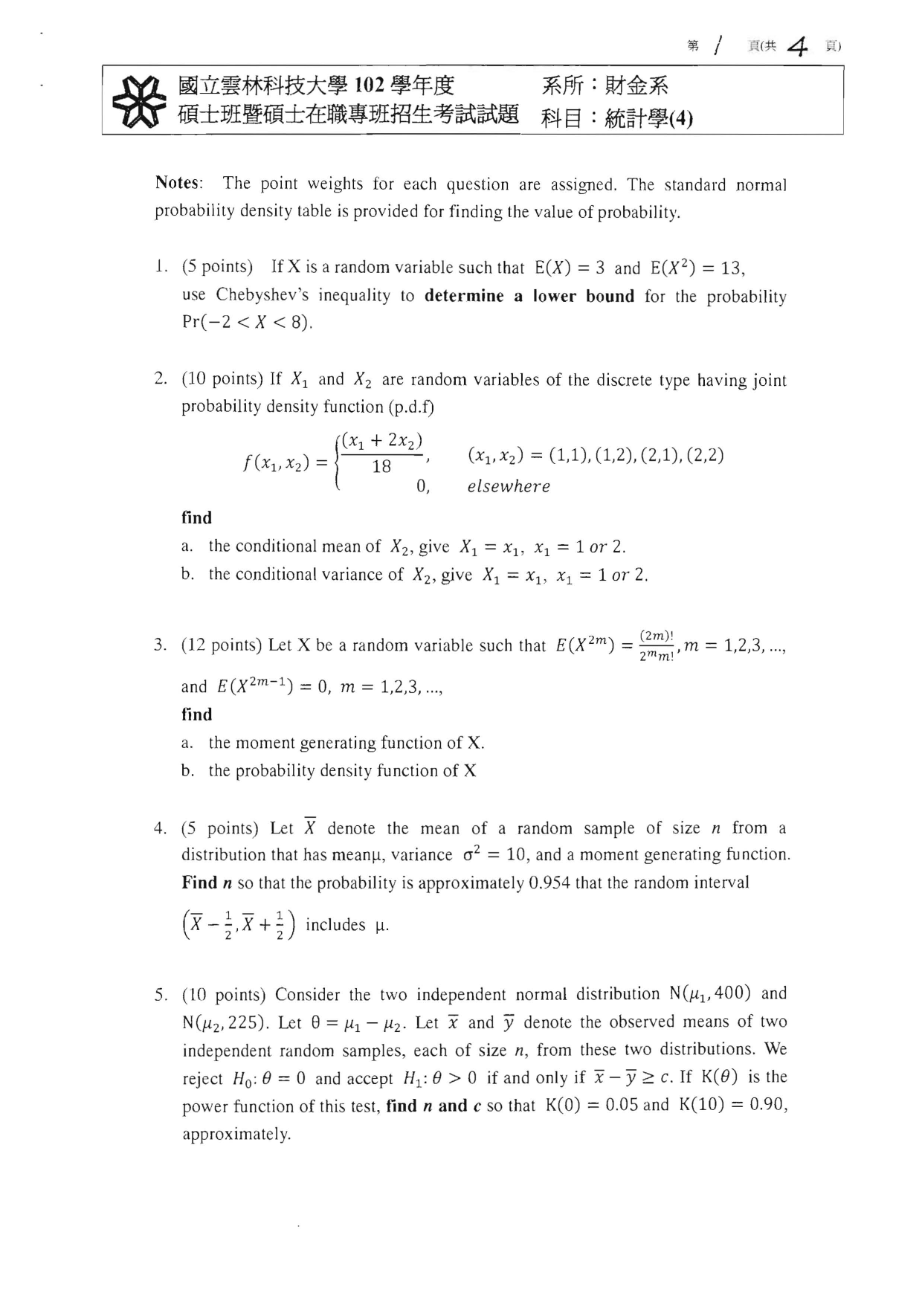
1 1

2

Https Www Math Wustl Edu Sawyer Hmhandouts Wishart Pdf

2
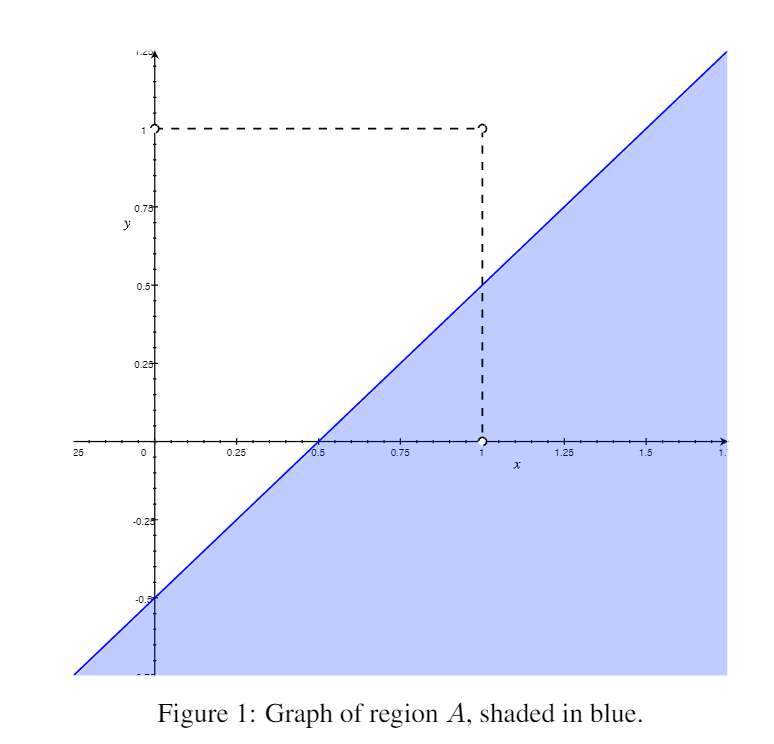
5 2 Joint Distributions Of Continuous Random Variables Statistics Libretexts

Https Encrypted Tbn0 Gstatic Com Images Q Tbn And9gcr25tef6v4nurws2kngeqzdif04iob3esypvergphtjedi 3mll Usqp Cau

Random Variable Wikipedia
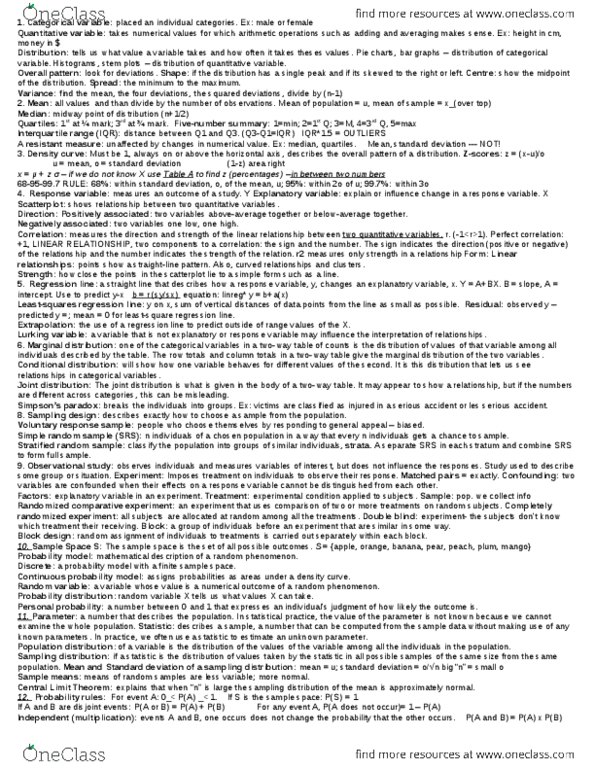
Stat 101 Study Guide Fall 2012 Final Simple Random Sample Squared Deviations From The Mean Joint Probability Distribution

Https Web Njit Edu Dhar Math244 Exiif2011 Sol Pdf
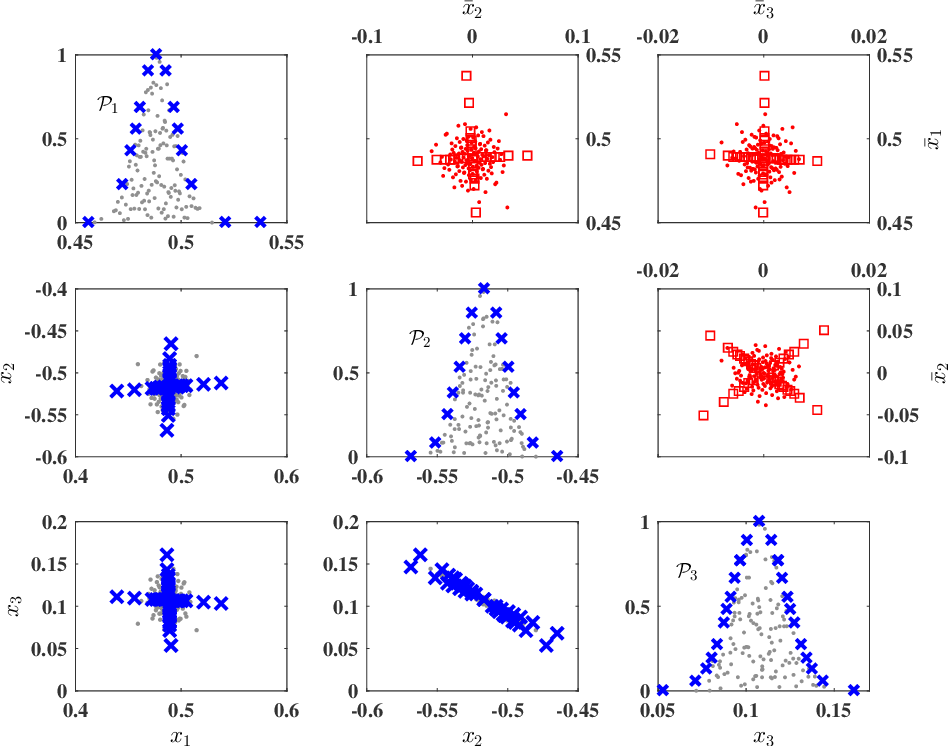
Pdf Random Sampling From Joint Probability Distributions Defined In A Bayesian Framework Semantic Scholar
Post a Comment for "Random Sample Joint Probability Distribution"